Being ahead of the curve is crucial in today's fast-paced and ever-evolving business landscape. Among the buzzwords that dominate discussions and headlines are "Artificial Intelligence" (AI) and "Machine Learning" (ML). While you may have a general understanding of these terms, there often needs to be more clarity regarding their definitions and how they relate.
This blog will explore the key differences and similarities between AI and ML, clarifying their distinct roles and exploring how these transformative technologies can revolutionize your business strategy. From streamlining operations to enhancing customer experiences, AI vs Machine Learning offers many opportunities for growth and innovation. By gaining a deeper understanding of their unique characteristics and applications, you can realize their potential to drive success in your business. So, let us take you on a tour of the dynamic world of AI and machine learning and show you how these technologies can help you achieve your business goals.
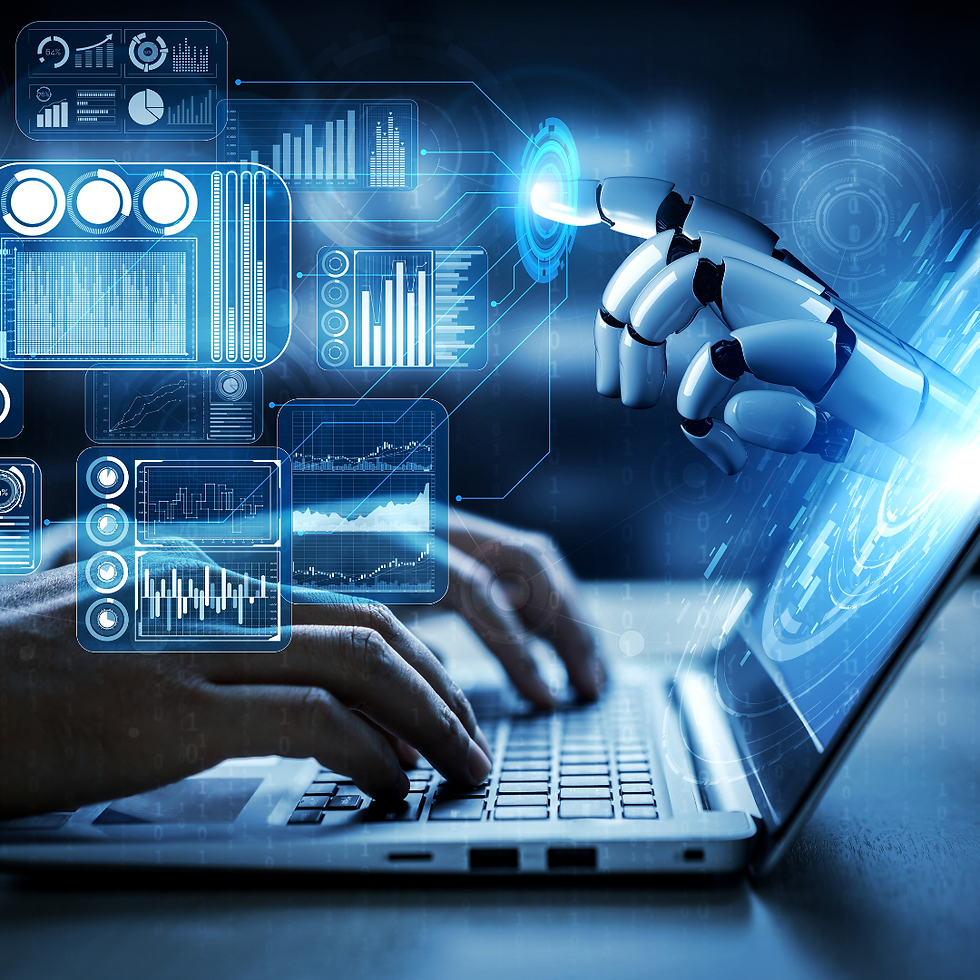
Let us first understand what Artificial Intelligence and Machine Learning is.
Artificial Intelligence: A Broad Concept
Artificial Intelligence simulates human intelligence in machines, helping them to perform tasks that generally require human intelligence. AI aims to create intelligent systems that can reason, learn, perceive, and make decisions as humans do. It encompasses various techniques, algorithms, and approaches that enable machines to exhibit intelligent behavior.
AI systems can be broadly categorized into Narrow AI (Weak AI) and General AI. Each type has different characteristics and applications.
Narrow AI, or Weak AI, refers to systems designed for well-defined tasks within a limited scope. These systems excel at executing predefined functions but cannot transfer knowledge from one domain to another.
Examples of narrow AI include voice assistants like Siri or Alexa, recommendation systems, and image recognition systems.
On the other hand, General AI, refers to AI systems with the skills to understand, learn, and apply knowledge across a wide range of tasks, similar to human intelligence. General AI represents a more comprehensive and advanced form of AI that is still largely hypothetical and under development.
Related: AI & Marketing Automation
Machine Learning: A Subset of AI
Machine Learning is a subset of AI. It focuses on developing algorithms and statistical models that allow computer systems to learn and improve from experience without being specifically programmed. In ML, machines are trained on large datasets to recollect patterns, make predictions, or perform specific tasks, based on basic statistical principles.
The core idea behind machine learning is to enable computers to learn from data and experience, adapt to new information, and improve their performance over time. It is achieved through algorithms that iteratively process data, extract meaningful patterns or representations, and update the system's internal parameters.
ML models can be broadly classified into 3 sections: supervised learning, unsupervised learning, and reinforcement learning.
Supervised learning involves training ML models with labeled data where the desired output or target is known. The model learns to map input data to the correct result by minimizing the error between its predictions and the known labels. This approach is commonly used in image classification, sentiment analysis, and speech recognition tasks.
Whereas unsupervised learning deals with unlabeled data, the model aims to discover underlying patterns or structures in the data. The main aim is to extract meaningful representations or groupings without direct guidance. Examples of unsupervised learning tasks include clustering, dimensionality reduction, and anomaly detection.
Reinforcement learning involves training an agent to communicate with an environment and learn through trial and error. The agent receives feedback in rewards or penalties based on its actions, supporting it to learn optimal behavior. Reinforcement learning has been successfully applied to game playing, robotics, and autonomous vehicle control tasks.
So the question arises: Is machine learning the same as artificial intelligence? If not, what is the relationship between them? Let us delve into this in the next section.
Relationship between AI and ML
The correlation between Artificial Intelligence and Machine Learning can be understood in terms of inclusivity. AI is a broader concept that addresses various methodologies, including ML. While ML is integral to AI, not all AI systems use ML techniques. AI involves other branches, such as expert systems, natural language processing (NLP), computer vision, and robotics.
ML can be considered a tool or technique within the broader range of AI. It provides the means for AI systems to learn and adapt based on data and experience, but it is not the only approach to achieving artificial intelligence.
In the next section, let us delve into the primary differences between AI and machine learning.
Key Differences between AI and ML
To understand deeply the key differences between AI and ML, let us examine their distinguishing characteristics:
Learning Capabilities:
Considering machine learning vs AI, ML focuses primarily on learning from data, while AI covers a broader range of capabilities, including reasoning, problem-solving, perception, and decision-making. AI systems aim to simulate human-like intelligence, while ML enables machines to learn patterns and make predictions based on data.
Explicit Programming:
Looking closer at AI vs machine learning, ML systems do not require specific programming for every task. Instead, they learn from data and adjust their behavior accordingly. AI systems, on the other hand, may involve explicit programming to perform specific tasks or simulate human-like behavior. ML algorithms can automatically extract patterns from data without defining any defined rules or logic.
Training vs. Inference:
A critical difference between AI and machine learning is that ML models require a training phase to learn patterns from labeled or unlabeled data. Once trained, they can perform inferences on new, unseen data, making predictions or decisions based on known patterns. However, AI systems may or may not involve a training phase, and a combination of learning and instructions typically determines their behavior.
Human-like Intelligence:
When comparing artificial intelligence and machine learning, AI aims to replicate human-like intelligence, enabling machines to exhibit behavior that resembles human cognitive abilities. ML, while not aiming for human-like intelligence, can allow devices to perform complex tasks by recognizing patterns in data.
Scope and Applications:
AI has a broader scope and finds applications in various domains, such as healthcare, finance, autonomous vehicles, and natural language processing. ML, a subset of AI, specifically focuses on developing algorithms for data analysis, predictions, and decision-making tasks. In contrast, ML techniques are widely used in fields such as image recognition, speech processing, fraud detection, and recommendation systems.
After exploring the key differences, let us examine the similarities between Artificial Intelligence (AI) and Machine Learning (ML).
Similarities Between AI and ML
Despite their differences, AI and ML have many similarities. Here are some of the similarities between AI and ML:
AI and ML aim to develop intelligent machines capable of performing tasks associated with human intelligence.
Both artificial intelligence and machine learning rely on data and algorithms to achieve their goals.
AI and ML are rapidly evolving fields with ongoing research and advancements.
Both AI and ML have a considerable impact on various industries, transforming processes and strengthening new applications.
AI and ML technologies are being implemented in a wide range of domains, from healthcare and finance to transportation and entertainment.
The progress of artificial intelligence and machine learning is driving innovation, creating new opportunities, and reshaping the way we interact with technology and the world around us.
Conclusion
Artificial intelligence and machine learning are used imprecisely, leading to confusion. However, it is essential to understand their differences, i.e., AI vs. Machine learning. AI is a broader concept comprising various techniques, methodologies, and applications, while ML is a specific subset of AI focused on learning from data. As technology advances, AI and ML will play increasingly significant roles in shaping our future. By understanding the differences and similarities between these two transformative technologies, we can better appreciate their distinctive contributions and maximize their potential to spark innovation in a wide range of industries. The synergy between artificial intelligence and machine learning holds the key to enabling intelligent systems that can transform various aspects of our lives, from healthcare and transportation to finance and entertainment. Adopting and exploring these technologies will undoubtedly lead to a more intelligent and automated future.
Comments